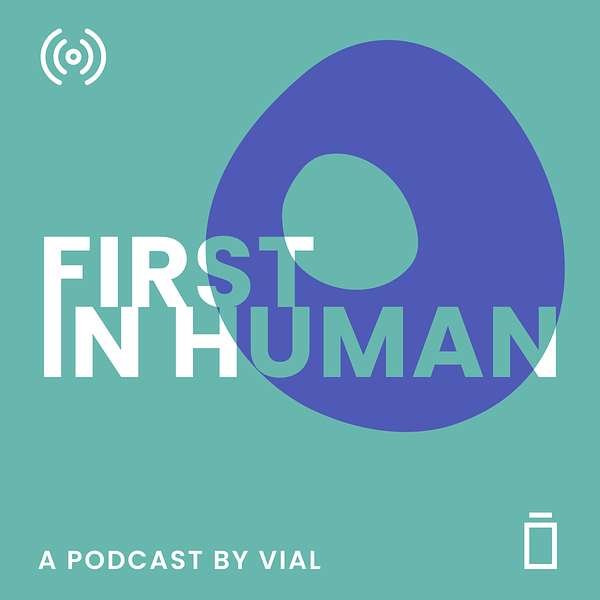
First In Human By Vial
First In Human is a biotech-focused podcast that interviews industry leaders and investors to learn about their journey to in-human clinical trials. Presented by Vial, a tech-enabled CRO with episodes launching weekly on Tuesday's.
🎧 Stay in the Loop!
For the latest news and updates, visit our website: https://vial.com
Follow us on social media for real-time insights:
Twitter: https://twitter.com/VialTrials
LinkedIn: https://www.linkedin.com/company/vialtrials
First In Human By Vial
Episode 39: Lucas Siow - CEO & Co-Founder at ProteinQure
Embark on a journey into the forefront of biotech with Lucas Siow, the visionary behind ProteinQure, as he unveils the impact of his computational platform on peptide therapeutics and delves into the art of team building. Explore the fusion of medicinal chemistry and high throughput screens, discover the essence of benchmarking in drug discovery, and gain insights into creating a versatile, learning-focused team in the biotech landscape from their Toronto base.
First In Human is a biotech-focused podcast that interviews industry leaders and investors to learn about their journey to in-human clinical trials. Presented by Vial, a tech-enabled CRO, hosted by Simon Burns, CEO & Co-Founder. Episodes launch weekly on Tuesdays. To view the full transcript of this episode, click here.
Interested in being featured as a guest on First In Human? Please reach out to catie@vial.com.
🎧 Stay in the Loop!
For the latest news and updates, visit our website: https://vial.com
Follow us on social media for real-time insights:
Twitter: https://twitter.com/VialTrials
LinkedIn: https://www.linkedin.com/company/vialtrials
You are listening to. First in Human, where we interview industry leaders and investors to learn about their journey to in-human clinical trials presented by Vile, the tech-enabled CRO hosted by Simon Burns, ceo and co-founder For Season 2, episode 1, we sit down with Lucas Siao, ceo and co-founder of Protein Cure. Stay tuned to learn how Protein Cure is advancing the creation of novel peptide therapeutics and what challenges they have faced in the world of drug discovery.
Speaker 2:Lucas, thank you for joining us on First in Human. Hi, Simon, Thanks for having us. So did I hear you're possibly a professional gambler in your first time. You're a very good poker player. You're also a system of data scientists. You're also a system of biologists. Take us through who you are. How did you come to be a tech founder? What background do you think makes for the focus area that you picked up?
Speaker 3:I'm one of four co-founders at Protein Cure. My academic training was on the applied math and statistics side. My first few jobs are more, I guess what's now called data science or machine learning. But Protein Cure is a company. First of all, we're using computational approaches to design specifically small protein therapeutics, so trying to design proteins that are far outside of nature and we can get into that but using a variety of different computational and experimental tools.
Speaker 3:Again, my background has always been on the applied math side.
Speaker 3:How do you use those kind of tools to help solve interesting and important problems?
Speaker 3:And I think when it comes to giving computational biology, it certainly influenced my thinking and as well as my co-founders who share similar backgrounds, though some of them are more on the software engineering side and some of them are more on the computational biology side. One of the big things that certainly I've taken from my background whether it's briefly spent time playing poker or briefly spent time betting on eSports in the early days of those things is really trying to let the results influence your design of your algorithms or to evaluation of your algorithms, and by that I mean it's important not to be results oriented. You're going to take some risks. Things will not work Things will work but at least trying to be very focused on having a good process and having a good focus on benchmarking and not falling in love with algorithms just because they're fancy, but not falling in love with just the latest and greatest because it's the latest and greatest, but really appreciating what is actually adding value to whatever your chosen problem is.
Speaker 2:Well, let's go one click deeper. I know you guys call your computational platform Pritching Studio. That's a bit interesting that you need aspects to that platform, and then you built your lab. What was that like? It gives the better sense of what you built.
Speaker 3:From day one, we've been really focused on again this special class of drugs known as peptide therapeutics. The most famous example would be something like Osempic, which today is very popular, though when we started the whole class itself was not super popular. And it's important because the way that this class of drug has always been discovered has two principal methods, which is still kind of the core of what we do at protein here. But we're just trying to do them in a computationally enhanced way. So if you think about the two pillars that we've built on the traditional way that these drugs were found things like insulin, things like Osempic was, they started with a human hormone and they would have medicinal chemists make mutations and try those mutations and experiments and see whether they were improving the properties. And in particular for these small proteins, you can go beyond the 20 natural amino acids so you can incorporate quite exotic building blocks, some of which are completely manmade and completely outside of nature, into your potential therapeutic. But the ability to do that in these small proteins is what creates such a vast chemical space. And we are using computational tools to predict the properties of these exotic peptides, but using very small data sets, because usually you only have maybe a 30 or 100 data points with that kind of information.
Speaker 3:And then the other reason that we've built our own wet lab is that the other common way to discover peptide therapeutics is through high throughput screens of displaying billions or even trillions of molecules, and that probably sounds like a lot, but it's actually a very small fraction of the potential chemical space.
Speaker 3:I wasn't sure many of your listeners would know. And so what we've done is we've tried to build in silico design libraries that you then make in the same traditional way whether that's phage or mRNA display, and you screen in a traditional way, but because every member is not just random, it's actually designed, hopefully you have much higher hit rates and each compound is going to be soluble, specific, well structured. Again, you still need that wet lab part, but it's the ability to make things better with the computational tools and also, for example, rank whether we think libraries will be effective against certain targets or not effective against certain targets, so we don't waste resources. That's been the approach to building something like Protein Studio, and combining all of these things and integrating many different pieces to model these exotic peptides has been the important and challenging part.
Speaker 2:It's interesting building a tech bar company that stays here to somehow simultaneously be a data science company really phenomenal. In building a team there, you have to be a robotics company, build out a lab and build a team that can be an operations driven company. If you do a lot of different things curious what do you find most challenging if you always surprisingly challenge it? What seemed like it was going to be obvious out of the gate, but it ended up being more challenging than it seemed.
Speaker 3:I think certainly in the computational space people are a little bit more generalists and you often think that you can hire somebody who knows, say, for example, programming language A, and they can find a way to do that. They can learn programming language B. Or somebody who maybe knows a certain flavor of neural networks, like computational networks, and they can learn some other approach, like transformers. You don't worry too much about people being super specialist.
Speaker 3:I think what has been a little bit surprising, at least to me, is how hard earned the secrets of bench scientists are. It's almost like a little bit of magic and art involved in knowing how an assay works, knowing exactly how to tweak things. It's not that it's impossible for someone to learn something, but usually you do need some expert or somebody who can teach. You can't just look up documentation, look up the method section of a paper and try to replicate. So that, certainly early on, quickly, was humbling and actually luckily, it was through our work with large pharma partners that we quickly learned that early in our history, so before we even were building anything, we kind of realized how much there was to know about the experimental side of things and even just like how do you dissolve your protein into solution, and how they're different from antibodies, all this different kind of stuff. So that was definitely a challenge that I think we didn't necessarily expect when we started, but we've adapted to as we've gone.
Speaker 2:I always talk about Canada. You guys are a big part of the Toronto biotech scene. First give me a curve how excited you are about the biotech scene and where you think it's going. But also you've seen it change quite a bit. A lot of companies have grown. Mars launch a couple of years ago. Things seem to be on an upper trajectory. Where have we come from? Where are we going in the Toronto biotech scene?
Speaker 3:The good news is that things are getting better. I think the bad news is that there's still just a long way to go. Toronto has a couple of companies that are doing, I think, reasonably well Things like Benchside, which makes it a little bit more adjacent to drug discovery, but still obviously in the life science space, companies like Deep Genomics and others have raised quite a bit of money to try to take their shot with their own computational approaches. Historically that wasn't even possible. There are companies like Adam Wise and others who moved to the US because they couldn't really build in Toronto. Still a lot of limitations in terms of one. You have to always convince everyone is, but especially investors, that this can be done here. Biotech is inherently an industry where people are always trying to de-risk and building outside of any of the hubs not just Canada, but outside of the Boston and San Francisco's of the world. You're always going to have some level of skepticism, but I think that is hopefully getting better.
Speaker 3:Toronto does have a large life science research expertise, just based in the universities. It's about getting that track record of success. It's funny 20 years ago people felt the same way about the software side of things, but then companies like Shopify and others, showed that you could build a world-class software company in Canada. Now it's just taken for granted. Nobody questions whether you could do that. Hopefully. Life science is on that track, though there are problems to solve like wet lab space and other things, but it certainly feels like they're getting better and the government and others are taking it more seriously. We're starting to see the core of a curging, just open the flagship office and actually two offices in Canada. Vancouver has a ton, so I think that Toronto and Canada in general are on the up.
Speaker 2:Hopefully Love to see it. The great North. Let's talk about your personal motivations. What keeps you going? You've been at this a long time. It's incredibly challenging running and building a startup. Usually there's some fire that's burning and you're going to keep motivated through all of it. What is it for you?
Speaker 3:It's funny.
Speaker 3:I don't know if this is going to be a typical answer, but I think me and my fellow co-founders we are really motivated by the intellectual challenge.
Speaker 3:This is still one of the most exciting spaces, in my opinion, if you're interested in really looking at how deep learning and all of these techniques are going to really impact the hardest problems.
Speaker 3:We're still at the earliest days of computational tools being applied to the understanding of biology, being applied to clinical trials, being applied to all of these different things. You get to wake up and learn about all of the most interesting problems, whether that's on the computational side or in the drug discovery side. You get to have a big impact if you solve those problems. If we are working whether it's with a partner on our own internal pipeline, on a disease that is a serious unmet need, or on even just understanding a certain mechanism, and we design something that unlocks that mechanism or elucidates it, it's easy to get excited about that every day and it's easy to get excited about applying cool technologies to hard problems. I think that has just never really changed, for me at least. I find it pretty easy to be motivated to continue to try to improve on the tools we've built and think about what are the kind of tools that are going to need to be built, because, again, to me, this is just the first innings.
Speaker 2:Obviously, we're clears out from AlphaFold. The AI advancements are coming in, pre-printed, every day, it seems. Where do you think we are in this century of biology it's something people will call it and how do you think the computational chemistry, computational biology approaches are going to evolve the next few years?
Speaker 3:Obviously there's a bias here, because we've taken a certain, I would say, philosophical perspective and we are trying to innovate along that perspective, to start high level. This is just the earliest days. We are going to look back at these tools and you're going to think of AlphaFold too, Like you look back and think of a 386 PC or something like that. You will realize that it was a breakthrough and a momentous one, but it will just be unrecognizable to the future things which the big part will be really incorporating physics extremely deeply into these models. And people already know that or have ideas on how to approach that, but of course it hasn't really come about yet, you know.
Speaker 3:I was just looking at it, like you said, there's 20 pre-prints every day now and this kind of broad space. There's multiple streams with all the top machine learning conferences, but I saw a recent one today where they were looking at small molecule docking and basically showing that the machine learning approaches create physically unrealistic poses. So basically they're not really accounting for what has to be happening behind the scenes in their predictions, and that can not necessarily a problem. There's still probably some value to it. But obviously going forward that's a lot of what Protinger has thought about is can we have a more 3D structure, physics inspired approach to doing the design of, in our case, specifically small proteins? But I think everyone is going to want to have that long term and it starts at a very small kind of system level. So just maybe a disease target and a ligand or the drug. But of course eventually you're going to want to simulate cells or simulate full pathways or something far more complex, and so there's just so much room to go in my mind.
Speaker 2:Last question for you. If you were speaking to yourself five or so years ago earlier on the company, or speaking to a tech buyer, founder looking to start a company today, what advice would you have for that?
Speaker 3:Again, I think it's a question that I think about a lot. There's a lot of founders. We try to think about what we could have done differently, or what we would tell ourselves, or how we would do it differently if we did it again. The one thing that is probably not that unusual, but just I always come back to as the core truth is that team is basically everything. You can't fall in love too much with technology. You should fall in love with the problem, hopefully, but in the end, it's going to be the team you hire that really determines things. I think, especially in tech bio, you're going to have to bridge many different functional areas, and so that requires a team that obviously can do the different functional areas, but also that can collaborate across them, which isn't always easy for specialists or for people who are even great in their particular area. You're going to have to adapt to technologies. When we first started some of the models for targets, we were using things like homology modeling. Alphafold was not even a thing, and then AlphaFold, too, became a thing and we had to be able to use it and had to be able to adapt quickly in terms of being able to generate disease target structures and understand how it worked and limitations and things like that. If you had hired for a very specific technology or a very specific tool set, you would be unable to adapt, and so it's about picking a team that can evolve quickly, which, again, for non-tech bio companies, that might be a little bit less necessary.
Speaker 3:If you can be confident in the tech stack that you're going to use and it's not going to change for reasonable periods of time, the path has been paved beforehand.
Speaker 3:Even things like the tech bio business model companies are constantly trying to iterate and constantly trying to learn from the failures and the successes which I'm sure you're doing as well in your own particular nation and trying to figure out how you can glean information from those who've come before and how you can iterate and improve.
Speaker 3:And again, that's a people thing. Like, I don't think you can necessarily hire the people who've been successful, but you try to hire people who can learn from those who were, or who can learn from the failures, and so that's the advice I always go back to is however much time you're spending on recruiting, or however much time you're spending on retention and your team in general, it's probably not enough and however much time you're spending on the other things. It's almost always worth trading off to try to improve, building the team better, or to having kind of the optimal team or as close as you can get. I think not that maybe this advice would be a little bit cliche, but it ends up just being the real truth when you think about it in my mind.
Speaker 2:Awesome. Thank you for joining us on First UnvEM. Thanks, Simon. Thanks for having me.
Speaker 1:Thanks for listening. Be sure to follow us on Spotify, apple, youtube and Google.